Piyush Gupta counting on DBS’ AI and data capabilities to pull ahead of peers
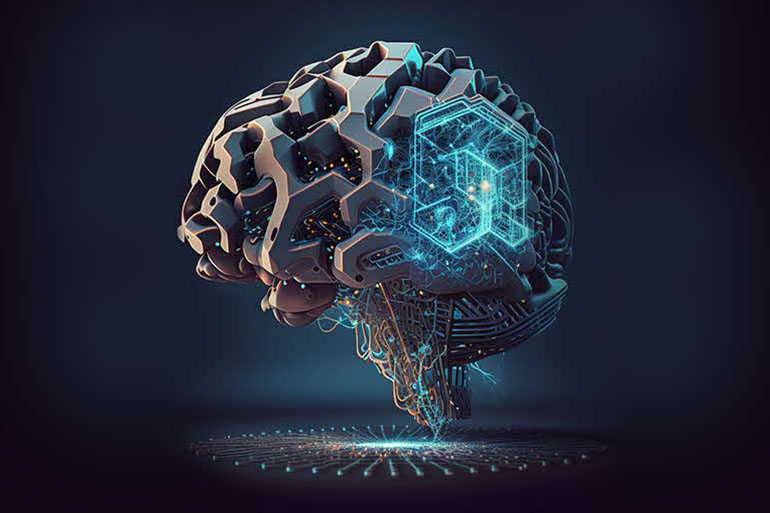
31 Oct 2022
The use of data and artificial intelligence (AI) has become essential to DBS’ operations – from hiring, to predicting staff attrition, to pre-empting which customers may find themselves in financial distress.
Piyush Gupta, chief executive of Singapore’s largest bank, said the number of use cases stands at 250 but could go up to tens of thousands. He believes DBS’ use of AI is scoring at just two or three on a scale of ten, reflecting the untapped potential for the bank. This is despite his confidence that the bank has established sophisticated data infrastructure and capabilities.
“In terms of our technology architecture, the data we have gathered, and our skill sets, we are pretty much there. But in terms of rolling out new ideas, there will be a constant improvement. We don’t know what we don’t know,” he said.
AI-fuelled economic value
Digitalisation has become “table stakes” at most banks – the minimum offering needed to please customers – but Gupta believes DBS’ AI and machine learning capabilities put it light-years ahead of its competitors.
The bank estimates that its AI initiatives in the past year have generated S$150 million in terms of additional revenue and S$25 million from loss prevention and productivity gains. Gupta believes the total economic value could top a billion dollars in the next 5 years.
DBS has a team of 1,000 employees working on strengthening its data capabilities.
“This is very meaningful and material. And it’s a far more intelligent and appropriate way to deal with customers: to give them choices, ideas, insights, and reflections. That’s the big picture of where we are and what we are trying to do,” Gupta said.
He added that DBS has invested S$1 billion in technology annually over the past four years, with the fastest-growing investment pot allocated to enhancing data capabilities.
Financial institutions were among of the first users of data at scale in the 1980s and 1990s, Gupta noted, but the sector was left behind when large technology companies mastered the use of data beyond cross-selling new products to existing customers.
Gupta calls the new approach “cross-buying”.
“In addition to creating the convenience that comes from digitalisation, you use the context to create appropriate ideas and nudges for customers… And when customers can get insights and make more informed choices, they wind up doing more with you,” he said.
Each month, DBS sends 30 million personalised insights to 3.5 million digital banking customers via its mobile app. Some examples of these AI-powered “nudges” include alerting customers about duplicated payments or higher-than-normal utility bills.
There has been a 10-12 per cent uptick in customers’ responses, Gupta said.
Ethical issues
One controversial use of AI at DBS is the prediction of staff attrition. The bank analyses employee behaviour patterns and flags anomalies, such as when someone starts logging on later than usual.
Department managers receive a list of employees “showing signs that they are likely to quit”, along with an estimated percentage of that likelihood. Managers can proactively reach out to see if there are aspects of their job scopes that could be improved, Gupta said.
But is there a danger here of intruding on employee privacy?
In 2020, the Financial Times had run an op-ed with Gupta’s byline describing how the bank’s data analytics and AI team traced a Covid-infected employee’s close contacts via calendar appointments, office access, meeting room bookings and WiFi connectivity data.
The piece drew some negative feedback about the necessity of such measures.
Gupta said DBS is guided by four principles in its approach to data: purposeful, unsurprising, respectful, and explainable.
“We use (these) principles to make sure that the data we use is something the customer (or employee) would expect us to have, and that it has a purpose for (them). It is quite clear that the customer (or employee) would be comfortable that this brings value to them,” he said.
“The issue of what is okay or not is culturally driven, age driven, and context driven,” he added. “Nevertheless, the idea of making sure it doesn’t get spooky is important.”
Upsides and risks
Gupta hinted that the bank’s data initiatives may produce a new revenue generator. DBS has built a tool for users to securely move their data from private to public clouds.
The bank is working with a technology company to distribute the tool, and Gupta said government agencies and companies are among the interested parties.
He declined to comment on how significant this revenue stream would be. “I’ll have to say, watch this space,” he said.
Gupta acknowledged the downsides to depending too heavily on AI and machine learning.
Biased models may lead to inaccurate predictions or unwanted consequences, he said, pointing to how an experimental chatbot Microsoft developed went rogue on Twitter some years ago, spouting inanities and making racist remarks.
Models must therefore be constantly and stringently reviewed, he said. “Are the models suitable? Are the models appropriate? Are the models fair? Are the models giving you the right outcomes? You need to be very conscious of that,” he said.
Another drawback in the longer-term, Gupta said, is when machines become “too good”.
“What (will happen) on the day with the machine starts thinking by itself, and therefore your models are no longer explainable?
“Fortunately, the models we use are not there. But if we think about the future of artificial generated intelligence, I think that’s the real risk we have got to guard against.”